Drug discovery is a complex task that often costs many millions of dollars through painstaking trial and error, but advanced computing technologies can dramatically accelerate the process. Now, a team of researchers from Cornell University have leveraged the supercomputing power of the Oak Ridge Leadership Computing Facility (OLCF) to use machine learning to improve drug design.
Machine learning and drug design historically haven’t gone hand-in-hand, as the massive datasets produced by molecular dynamics are often complex and largely irrelevant to drug performance. “Machine learning has never been used to classify these mechanisms for drug design—and certainly not at the scales we’re dealing with,” said Harel Weinstein, director of the Institute for Computational Biomedicine at Cornell, in an interview with OLCF. “At the beginning of this study, the question was: how do you even present this data to a machine learning algorithm?”
Weinstein approached one of his PhD students, Ambrose Plante, with this question. Plante decided to reformat the data into a picture to make it more easily interpretable for an ML algorithm, assigning a pixel for each atom. He then tasked the algorithm with analyzing those images to assess functional selectivity (the manner in which a drug bonds to specific proteins, forcing the proteins to send signals).
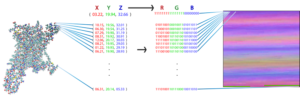
Understanding how to affect the protein in a way that it sends different signals is crucial to drug development, particularly when it comes to the G protein-coupled receptors (GCPRs) targeted by the majority of drugs on the market today. “These are the most popular receptors, in terms of the pharmacopeia,” Weinstein said. “The research is special because of the method by which these interactions were analyzed.”
To convert the 3D data into 2D images and train the neural network to understand these signaling pathways, the researchers turned to OLCF’s Titan supercomputer after receiving a time allocation through the Innovative and Novel Computational Impact on Theory and Experiment (INCITE) program. Titan, a system with 18,688 AMD Opteron CPUs and 18,688 Nvidia K20 GPUs that delivered nearly 18 Linpack petaflops, was decommissioned last August.
The researchers found that the neural network was highly accurate, and are now turning to more powerful and complex applications of the same strategy. With Titan decommissioned, however, they are now turning to OLCF’s Summit supercomputer. With 4,608 nodes (each holding two IBM Power9 CPUs and six Nvidia Volta GPUs), Summit delivers 148 Linpack petaflops and currently stands as the most powerful publicly-ranked supercomputer.
“Not only could this help us understand and potentially mitigate things like drug addiction, but it will allow us to look at drug design from a completely different point of view and with more specific and hence, more powerful criteria,” Weinstein said.
About the research
The research discussed in this article was published as “A Machine Learning Approach for the Discovery of Ligand-Specific Functional Mechanisms of GPCRs” in Molecules. It was written by Ambrose Palnte, Derek M. Shore, Giulia Morra, George Khelashvili and Harel Weinstein and can be accessed here.
To read the original article by OLCF’s Rachel Harken, click here.