March 19, 2024 — What happens when Department of Energy (DOE) researchers join forces with chemists and biologists at the National Cancer Institute (NCI)? They use the most advanced high-performance computers to study cancer at the molecular, cellular and population levels. The results offer insights into cancer and accelerate advances in precision oncology and scientific computing.
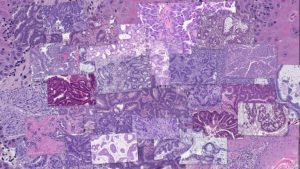
“Working with people who are super-passionate about solving cancer brings an entirely new type of energy and motivation into the collaboration,” said Rick Stevens, Argonne National Laboratory’s associate laboratory director for the Computing, Environment, and Life Sciences Directorate.
The DOE-NCI collaboration, which is part of the Cancer Moonshot, began in 2016 and encompasses three projects: AI-Driven Multiscale Investigation of the RAS/RAF Activation Lifecycle (ADMIRRAL); Innovative Methodologies and New Data for Predictive Oncology Model Evaluation (IMPROVE); and Modeling Outcomes Using Surveillance Data and Scalable AI for Cancer (MOSSAIC).
MOSSAIC automated the analysis and extraction of information from millions of cancer-patient records to help determine optimal cancer-treatment strategies across a range of patient lifestyles, environmental exposures, cancer types and healthcare systems.
ADMIRRAL’s goal was to better understand the RAS oncogenic signaling system. RAS is a protein embedded in the surface of every cell and switches on and off to send signals to the cell’s interior. This system is a root cause of about 40% of cancers when it gets stuck in the on position. So the researchers are using large-scale computing to build a molecular dynamics model of the protein. They are creating a simulation by applying models ranging from the size of a single cell to the scale of the molecules and their individual atoms. AI can then use the simulations to help discover the intricacies of how RAS works.
IMPROVE developed a framework to compare and evaluate computer models designed to predict drug response, optimize drug screening, and drive precision medicine for cancer patients. These promising models are based on deep learning, a type of machine learning that mimics the brain’s ability to recognize complex patterns and yield accurate predictions from large inputs of raw data.
The DOE’s Exascale Computing Project (ECP) plays a key role in all three of these ventures.
CANDLE Ties It All Together
In particular, these DOE-NCI endeavors are supported by the ECP’s CANcer Distributed Learning Environment (CANDLE) project, which deploys a scalable deep neural network code to exascale computers that can handle more than a million trillion calculations per second.
To create this infrastructure, Stevens, leader of IMPROVE, and colleagues moved AI tools to the exascale platform to build a software environment that enables working on these three projects without duplication of effort across the laboratories. “The Exascale Computing Project achieved great speedups on exascale hardware — it was amazingly successful,” he said.
CANDLE, which wrapped up at the end of 2023, started out as a bit of a computer science project. “We worked on the tools, the libraries for the exascale environment, and dealt with chip and machine performance,” Stevens explains. “Techniques we developed are quite useful for AI and other problems beyond cancer, such as making headway on problems in materials science and gaining a better understanding of COVID-19.”
As the core deep-learning software system underlying multiple DOE-NCI projects, “CANDLE truly enabled open-minded thinking when considering whether machine learning or deep learning is a possibility for a given challenge,” said Eric Stahlberg, director of biomedical informatics and data science at the Frederick National Laboratory for Cancer Research (FNLCR), which joined the collaboration on behalf of NCI to launch CANDLE.
At a technical level, FNLCR and NCI contributed to the early development and direction of CANDLE, ensuring its software would be usable for the broader biomedical research community via developing workshops and supporting training, and bringing essential data to the collaboration to drive development and innovative applications to cancer research.
CANDLE has changed thinking about how to approach cancer drug discovery using data from multiple sources. It also has supported essential research in RAS-related cancers — helping to bridge understanding and experimental observations across different time and size scales. “Its deep-learning models also boost the efficiency of information extraction from patient data to improve the available cancer surveillance research information,” Stahlberg said.
Improving Cancer Drug Discovery
Ultimately, ADMIRRAL and IMPROVE researchers intend to boost cancer drug discovery. If researchers can understand how RAS works or sequence a tumor’s RNA and DNA, they can work to predict drugs with the potential to impact the RAS system.
In a precursor to IMPROVE, researchers compiled decades’ worth of data about known tumors, the drugs used to treat them and their outcomes. “We built machine-learning models to represent both the tumor and the drug to predict the response of the tumor to a given drug,” Stevens said. “The idea was to use them in preclinical experiments to explore new drugs and try to better understand the biology of the tumor — whether it responds or not to the new drug.”
IMPROVE’s approach is closely related to the concept of precision medicine, where patients get customized treatments based on the genetics of their tumors. “We did this for about five years, and were quite successful building these models,” Stevens commented.
Then, the AI community started building lots of similar types of models. “A few years ago, we decided rather than continuing to push on the model itself, we needed to build a system to allow us to compare and benchmark these models against each other, because there are so many of them,” Stevens said. “There are now more than 100 models from groups that are more or less aiming to predict the same thing, so the problem has shifted a bit to being able to understand which models are better for certain tumors or classes of drugs.”
The team discovered that no single way of representing drugs emerged as dominant. “Our representations of drugs had strengths and weaknesses, so we started combining the representations,” Stevens explains. “We did the same thing with characterizing tumors, and at first we thought mutation data would be the most useful, but it turns out that genetic-expression data was the most predictive or informative.” That makes sense given that a gene — mutated or not — creates an impact only if it’s turned on.
This work “provides a way for the community to commonly evaluate models and share insights on data needed to improve these models,” Stahlberg said. “As a result of the IMPROVE project, the collective contributions of scientists into this problem are being brought together, harmonized and compared, which provides greater insight to benefit the community as a whole.”
Modernizing National Cancer Surveillance
ECP’s CANDLE is helping to speed and modernize national cancer surveillance as part of the MOSSAIC project. The team developed and deployed novel deep-learning, natural-language-processing solutions to rapidly screen and extract clinical information from unstructured clinical text documents, including pathology and radiology reports.
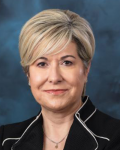
CANDLE MOSSAIC is now used by 12 Surveillance, Epidemiology, and End-Results (SEER) registries, where it “scans reports 18,000 times faster than cancer registrars,” said Gina Tourassi, associate lab director, Computing and Computational Sciences Directorate, Oak Ridge National Laboratory (ORNL). She led MOSSAIC with ORNL’s Heidi Hanson, group lead of the biostatistics and biomedical informatics group.
“The model auto-coded 20% of the cases with more than 98% accuracy — saving 7,800 hours of manual screening,” Tourassi added. “This level of performance paves the way for a modernized national cancer surveillance program to achieve near-real time cancer incidence reporting — a process that currently takes 22 months.”
Because this work is part of a translational project and meant to be fully developed for use by research and medical professionals, it presented distinct challenges. The team’s mission was to deliver end-to-end science, identifying existing data sources and tapping into them, evaluating the data, designing the computer models, and delivering AI-based solutions that users could deploy.
Tourassi is “proud that we delivered our milestones — demonstrating the power of transdisciplinary science enabled by world-class computing resources and domain experts that live at the bleeding edge of computational science.”
Cross-Agency Cooperation for the Win
All four of these projects show the power of DOE-NCI cross-agency teamwork.
Early on, the teams spent a lot of time learning the most advanced science and languages, plus new ways of talking to others outside their fields. “This happens in other interdisciplinary collaborations but was particularly necessary here,” Stevens said. “And having partners from NCI allows the computing people to decode how we can help a lot faster.”
The interagency collaboration forced everyone to challenge the status quo within their own fields. “For NCI,” Tourassi said, “the project challenged the notion that deep learning isn’t appropriate for clinical language processing.”
Six years ago, some at NCI were skeptical when CANDLE introduced deep learning for automated information capture from clinical reports to the national surveillance program.
“For DOE, there was skepticism about natural-language processing being an exascale problem,” Tourassi said. “Fast forward to now. Large language models are the posterchild of AI applications on exascale computing platforms. Both agencies were able to advance our respective missions and deliver scientific breakthroughs with lasting value.”
CANDLE is “a tremendous example of team science — working together and sharing insights from multiple domains to create and improve results,” Stahlberg said. “Its capabilities have been guided by real problems to address real needs.”
Source: Sally Johnson, ECP