Declining DNA sequencing costs and the rush to do whole genome sequencing (WGS) of large cohort populations – think 5000 subjects now, but many more thousands soon – presents a formidable computational challenge to researchers attempting to make sense of large cohort datasets. No single architecture is best. This month researchers report developing a hybrid approach that combines cloud (AWS), local high performance compute (LHPC) clusters, and supercomputers.
Their fascinating paper, A hybrid computational strategy to address WGS variant analysis in >5000 samples, spells out in some detail the obstacles associated with using each resource and how to divide the work to maximize throughput and minimize cost. Computational resources used included: Amazon AWS; a 4000-core in-house cluster at Baylor College of Medicine; IBM power PC Blue BioU at Rice University and Rhea at Oak Ridge National Laboratory (ORNL). DNAnexus was also a collaborator.
“Large cohort studies,” write the authors, “are extremely useful for discovering genotype phenotype associations and to characterize variation with great public health significance. The decreasing costs of sequencing are increasingly making it possible to sequence whole genomes in the millions in the coming years. The past decade has also seen the development of many joint calling approaches for genomic data produced with low coverage whole genome sequencing. Joint calling is necessary for low to medium coverage sequencing projects (~10×) as it further reduces false positives rate especially at the rarer end of the site frequency spectrum.”
The multidisciplinary team, led by Baylor, developed a genomics analysis pipeline – goSNAP – that distributes the workflow across the platforms. As a proof of principle, analysis was performed of Cohorts for Heart And Aging Research in Genomic Epidemiology (CHARGE) WGS freeze 3 dataset in which joint calling, imputation and phasing of over 5300 whole genome samples was produced in under six weeks using four state-of-the-art callers (SNPTools, GATK-HaplotypeCaller, GATK-UnifiedGenotyper, and GotCloud.)
“The entire operation was finished in 50 days with a total core hour usage of ~ 5.2 million across all the infrastructures. Each aligned BAM file was split into 1 Mbp region for joint calling on AWS. This created a cache data footprint of 360 TB with a time to live not exceeding 14 days. Only 6 TB of data was transferred across all platforms. The goSNAP pipeline is designed to minimize egress charges, data storage charges and data transfer costs. It optimizes on concurrent core usage to be cost effective and fast. To the best of our knowledge, ensemble calling on a WGS cohort with over 5000 samples has not been done before and this approach can be easily scaled to 10,000 samples.”
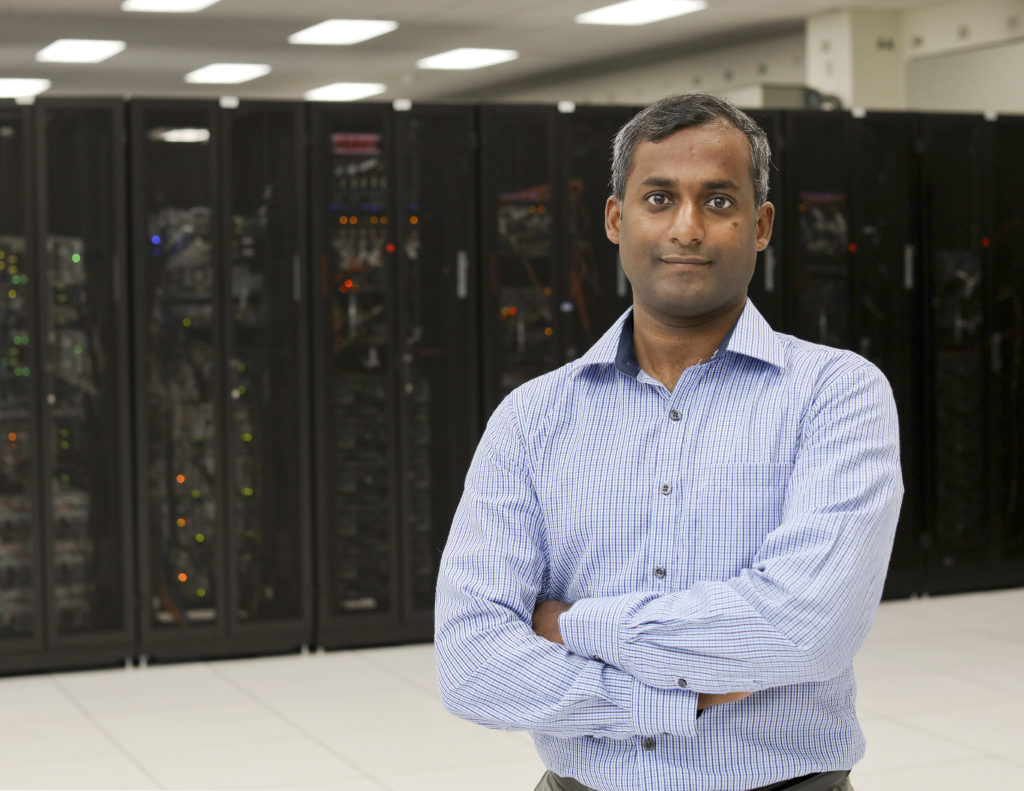
“This is an excellent example of two scientific communities coming together to address challenging science problems. We are happy to have played a part in conducting the analysis of such unprecedented scale,” said Manjunath Gorentla Venkata, co-author and ORNL computer scientist in an account of the work on the ORNL website. “While researchers from Baylor discussed the problem, we did not have a ready-made solution. After multiple discussions, we were convinced that mapping pipeline components based on system architecture strengths and tailoring parameters to the architecture would provide quality analysis with a relatively short turnaround.”
“There was previously no infrastructure for this large of a set, at 5,000 samples,” said Dr. Eric Boerwinkle, associate director of Baylor’s Human Genome Sequencing Center and dean of UT Health School of Public Health. “To address this, we employed a combination of platforms to perform large-scale variant calling, while maintaining high quality data.”
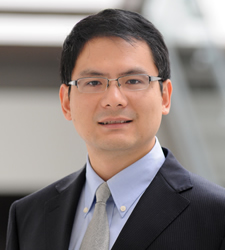
Their work, report the authors, demonstrates variant calling pipelines using a hybrid computational environment can leverage the strengths of each architecture to process cohorts with thousands of whole genome samples in real-time while minimizing operational costs.
The specifics of how the workflow (variant site identification; consensus site filtering step; genotype likelihood; and imputation & phasing) is divided up among the computational resources are best gleaned directly from the paper as some steps overlap. The authors write,” There has been some past work on porting state-of-the-art variant calling pipelines for targeted whole exome sequencing of thousands of samples to the Amazon Web Services (AWS) cloud, but a cloud based ensemble calling workflow for thousands of whole genomes is lacking.”
More broadly the authors note the following issues with each class of infrastructure:
- Most LHPCs with typical research environments have few PBs of storage and millions of core-hours per month and are constrained by hardware limits on data storage, computing power and data transfer bandwidth to carry out large computes.
- Scalability is not a problem for the AWS computing environment as it allows flexibility to increases the compute and data resources with a ‘pay per use’ model. However, the outbound data transfers incurs a cost which scales linearly with the amount of data transferred. It is also necessary to optimize on all aspects of the compute including memory bandwidth and capacity (RAM), computing cores (CPU) and IO capacity and bandwidth (HDD) to make optimal use of the instances and achieve cost-effectiveness. For projects involving big data, there is an additional cost of implementing data parallelization to overcome the limitations of local instance on HDD space.
- The large supercomputing infrastructure has an extremely large data store, premium hardware optimized for high IO bandwidth, low-latency and high bandwidth network, and dedicated hardware and software support for CPU-intensive operations, but computing jobs have to finish within hard wall time limits. (For example, Titan at ORNL requires all jobs to finish within 24 hrs. Scheduling delays in allocating large number of resources can add to the turnaround times.)
Click on the image below to get a better sense of how the computational were used in this study.
The team used the Rhea computing cluster at the Oak Ridge Leadership Computing Facility to reconstruct chromosomal segments inherited from parents and to statistically predict the makeup of incomplete or missing genetic sequences from discovered genetic markers. This step was the most computationally intensive and required the greatest amount of power to calculate the probabilities of the most likely genetic patterns. More than 75 percent of this step was finished on Rhea and the rest was completed on supercomputers at Rice University. Baylor utilized the Amazon Web Services cloud computing environment to store raw data and discover genetic variants across the thousands of genome samples.
The authors conclude:
“With increasing number of genomic datasets freely available on the AWS cloud, the next generation of variant calling pipelines will also be increasingly common in the AWS environment. While the costs of storage and compute cores in the AWS environment is declining, it may still be prohibitively costly to carry out many steps of standard variant calling workflow on the cloud. A hybrid computational approach involving multiple HPC systems may be an important future direction to explore. Our work on the goSNAP pipeline demonstrates that using a hybrid computation strategy can be cost effective and fast even with thousands of individual genomes.”
Link to ORNL article:
Link to Baylor article:
https://www.bcm.edu/news/genome-sequencing/new-scalable-whole-genome-data-analysis
Link to paper on open access publisher BioMed Central (Sep 10, 2016,) https://bmcbioinformatics.biomedcentral.com/articles/10.1186/s12859-016-1211-6