In November 2015, three colleagues representing the US Department of Energy (DOE) Office of Science’s three major supercomputing facilities struck up a conversation with a science and technology book publisher about a project to prepare a publication focusing on the future of application development in anticipation of pre-exascale and exascale supercomputers and the challenges posed by such systems.
Two years later, the fruits of that discussion became tangible in the form of a new book, which debuted at SC17. Exascale Scientific Applications: Scalability and Performance Portability captures programming strategies being used by leading experts across a wide spectrum of scientific domains to prepare for future high-performance computing (HPC) resources. The book’s initial collaborators and eventual coeditors are Tjerk Straatsma, Scientific Computing Group leader at the Oak Ridge Leadership Computing Facility (OLCF); Katerina Antypas, Data Department Head at the National Energy Research Scientific Computing Center (NERSC); and Timothy Williams, Deputy Director of Science at the Argonne Leadership Computing Facility (ALCF).
Twenty-four teams, including many currently participating in early science programs at the OLCF, ALCF, and NERSC, contributed chapters on preparing codes for next-generation supercomputers, in which they summarized approaches to make applications performance portable and to develop applications that align with trends in supercomputing technology and architectures.
In this interview, Straatsma, Antypas, and Williams discuss the significance of proactive application development and the benefits this work portends for the scientific community.
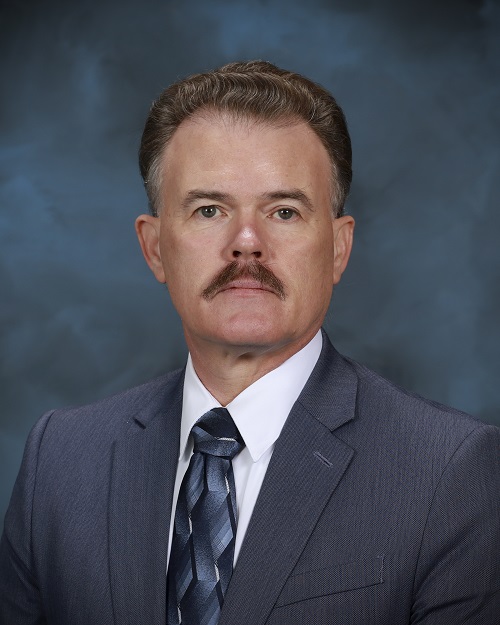
How did this book come to be written?
Tjerk Straatsma: When we proposed writing the book, the intent was to provide application developers with an opportunity to share what they are doing today to take advantage of pre-exascale machines. These are the people doing the actual porting and optimization work. Through their examples, we hope that others will be inspired and get ideas about how to approach similar problems for their applications to do more and better science.
For quite some time, the three DOE ASCR [Advanced Scientific Computing Research] supercomputing facilities have been the leaders when it comes to working on performance portability for science applications. For our users, it’s very important that they can move from one system to another and continue their research at different facilities. That’s why DOE is very much interested in the whole aspect of portability—not just architectural portability but also performance portability. You want high performance on more than just a single system.
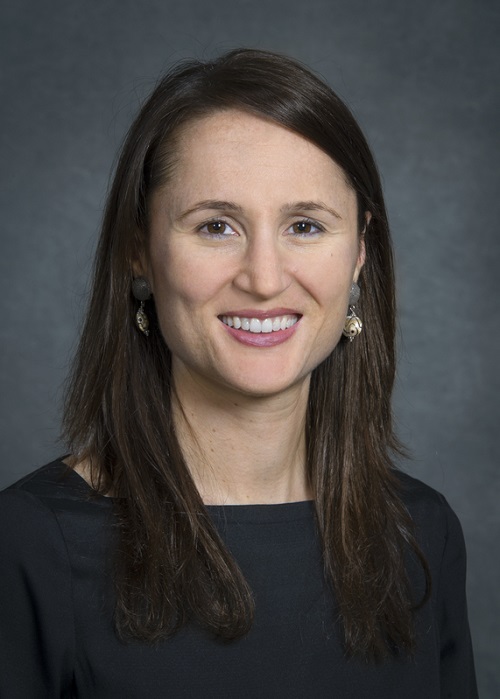
Katerina Antypas: As the three of us discussed the different application readiness programs within our centers, it was clear that despite architectural differences between the systems at each center, the strategies to optimize applications for pre-exascale systems were quite similar. Sure, if a system has a GPU, a different semantic might be needed, but the processes of finding hot spots in codes, increasing data locality, and improving thread scalability were the same. And in fact, teams from NERSC, OLCF, and ALCF talked regularly about best practices and lessons learned preparing applications. We thought these lessons learned and case studies should be shared more broadly with the rest of the scientific computing community.
Timothy Williams: Nothing instructs the developer of scientific applications more clearly than an example. Capturing the efforts of our book’s authors as examples was an idea that resonated with us. Measuring and understanding the performance of applications at large scale is key for those developers, so we were glad we could include discussions about some of the tools that make that possible across multiple system architectures. Libraries supporting functions common to many applications, such as linear algebra, are an ideal approach to performance portability, so it made good sense to us to include this as a topic as well.
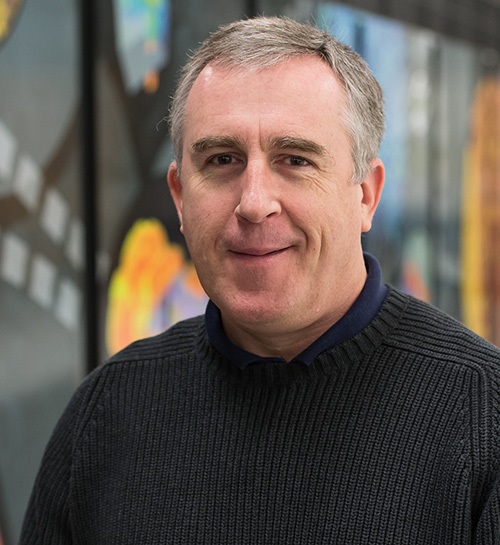
Why is it important for these programming strategies to be shared now?
Straatsma: It’s important because DOE’s newest set of machines is starting to arrive. In 2016, NERSC delivered Cori, which comprises 9,688 Intel Xeon Phi Knights Landing processors, each with 68 cores. As we speak, the OLCF is building Summit—which will be around eight times more powerful than our current system, Titan, when it debuts in 2018. The ALCF is working to get its first exascale machine, Aurora, and the OLCF and NERSC are already working on the machines to follow their newest systems, at least one of which is likely to be an exascale machine.
It takes a long time to prepare codes for these new machines because they are becoming more and more complex. Hierarchies of processing elements, memory space, and communication networks are becoming more complex. Effectively using these resources requires significant effort porting applications. If you do that in a way that makes them portable between current machines, there’s a better chance that they will also be portable to future machines—even if you don’t know exactly what those systems will look like.
This is what this book is all about: providing a set of practical approaches that are currently being used by application development teams with the goal of getting applications to run effectively on future-generation architectures.
Antypas: There are three key technologies that applications need to take advantage of to achieve good performance on exascale systems: longer vector units, high bandwidth memory, and many low-powered cores. Regardless of vendor or specific architecture, future exascale systems will all have these features. The pre-exascale systems being deployed today—Cori at NERSC, Theta at ALCF, and Summit at OLCF—have early instances of exascale technologies that scientists can use to optimize their applications for the coming exascale architectures. Preparing applications for these changes now means better performing codes today and a smoother transition to exascale systems tomorrow.
Williams: Exascale computing is coming to the US in an accelerated timeframe—by 2021. This makes the work on applications, tools, and libraries documented in this book all the more relevant. Today is also a time of extraordinary innovation in both hardware and software technologies. Developing applications that are up to today’s state of the art, and well-positioned to adapt to those new technologies, is effort well spent.
What other major challenges are science and engineering application developers grappling with?
Straatsma: The biggest challenge is expressing parallelism across millions and millions—if not billions—of compute elements. That’s an algorithmic challenge. Then you have the hardware challenge, mapping those algorithms on to the specific hardware that you are targeting. Whether you have NVIDIA GPUs as accelerators together with IBM Power CPUs like on Summit or you’re looking at NERSC’s Cori system with its Intel Knights Landing processors, the basic story is the same: Taking the parallelism you’ve expressed and mapping it on to that hardware.
It’s a tall order, but, if done right, there is an enormous payoff because things that are being developed for these large pre-exascale machines tend to also lead to more efficient use of traditional architectures. In that sense, we’re at the forefront of the hardware with these machines, but we’re also at the forefront of the software. The benefits trickle down to the wider community.
Antypas: Besides the challenges associated with expressing on-node parallelism and improving data locality, scientists are grappling with the huge influx of data from experiments and observational facilities such as light sources, telescopes, sensors, and detectors, and how to incorporate data from these experiments into models and simulations. In the not too distant past, workflows started and ended within a supercomputing facility. Now, many user workflows start from outside of a computing facility and end with users needing to share data with a large collaboration. Data transfer, management, search, analysis, and curation have become large challenges for users.
Williams: Whether you view it as a challenge or an opportunity is a matter of perspective, but those developers who are themselves computational scientists are now more tightly coupled to the work of experimentalists and theorists. They are increasingly codependent. For example, cosmological simulations inform observational scientists of specific signs to look for in sky surveys, given an assumed set of parameter values for theoretical models. Particle-collider event simulations inform detectors at the experiment about what to look for, and what to ignore, in the search for rare particles—before the experiment is run.
How is scientific application development, which has traditionally entailed modeling and simulation, being influenced by data-driven discovery and artificial intelligence?
Straatsma: Most of the applications that we have in our current application readiness programs at the DOE computing facilities use traditional modeling and simulation, but artificial intelligence, machine learning, and deep learning are rapidly affecting the way we do computational science. Because of growth in datasets, it’s now possible to use these big machines to analyze data to discover underlying models. This is the broad area of data analytics. In our book, one such project is using seismic data analysis to derive models that are being used to get a better understanding of the Earth’s crust and interior.
In a sense, it’s doing computational science from the opposite direction than what has traditionally been done. Instead of having a model and simulating that model to create a lot of data that you use to learn things from your system, you start with potentially massive datasets—experimental or observational—and use inference methods to derive models, networks, or other features of interest.
Antypas: Machine learning and deep learning have revolutionized many fields already and are increasingly being used by NERSC users to solve science challenges important to the mission of the Department of Energy’s Office of Science. As part of a requirements-gathering process with the user community, scientists from every field represented noted they were exploring new methods for data analysis, including machine learning. We also expect scientists will begin to incorporate the inference step of learning directly into simulations.
Williams: Computational scientists now increasingly employ data-driven and machine learning approaches to answer the same science and engineering questions addressed by simulation. Fundamental-principles–based simulation and machine learning have some similarities. They can both address problems where there is no good, high-level theory to explain phenomena. For example, behavior of materials at the nanoscale, where conventional theories don’t apply, can be understood either by simulating the materials atom-by-atom or by using machine learning approaches to generate reduced models that predict behavior.
In the foreword, the contributors to this book are referred to as “the pioneers who will explore the exascale frontier.” How will their work benefit the larger scientific community?
Straatsma: In multiple ways. The most obvious benefit is that we get a set of applications that run very well on very large machines. If these are applications used by broad scientific communities, many researchers will benefit from them. The second benefit is in finding methodologies that can be translated to other codes or other application domains and be used to make these applications run very well on these new architectures. A third benefit is that application developers get a lot of experience doing this kind of work, and based on that experience, we have better ideas on how to approach the process of application readiness and performance portability.
Williams: With each step forward in large-scale parallel computing, a cohort of young scientists comes along for the ride, engaged in these pioneering efforts. The scale of this computing, and the sophistication of the software techniques employed, will become routine for them going forward. This is really just a manifestation of the advance of science, which builds on successes and corrects itself to be consistent with what we learn.
After coediting this volume, are there any key lessons that you hope readers take from this work?
Straatsma: I hope that people who are wondering about HPC at the scale we’re talking about will get inspired to think about what these future resources could do for their science or think bigger than what they’re thinking now. To draw one example from the book, astrophysicists are developing techniques for exascale systems that are projected to enable simulation of supernova explosions that include significantly larger kinetic networks than can be used today, and these systems can do this faster and more accurately. That’s just one example of the many described in this publication of exascale-capable applications with the promise of enabling computational science with more accurate models and fewer approximations, leading to more reliable predictions.
Oak Ridge National Laboratory is supported by the US Department of Energy’s Office of Science. The single largest supporter of basic research in the physical sciences in the United States, the Office of Science is working to address some of the most pressing challenges of our time. For more information, please visit science.energy.gov.
Jonathan Hines is a science writer at Oak Ridge National Laboratory.