The Quantum Economic Development Consortium last week released a new paper on benchmarking – Quantum Algorithm Exploration using Application-Oriented Performance Benchmarks – that builds on earlier work and is an effort to “broaden the relevance and coverage” of the QEDC benchmark suite.
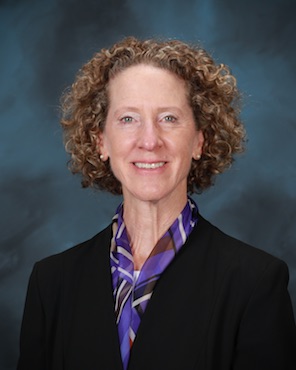
Celia Merzbacher, executive director of QED-C, told HPCwire, “QED-C continues to expand and refine the suite of publicly available benchmark programs aimed at measuring performance and progress of quantum computers based on execution of key fundamental algorithms. These tools were developed by leading experts from a cross-section of QED-C members with assistance from nine student interns over the last three years. Stay tuned for further additions and improvements.”
Benchmarking efforts aren’t new. Indeed, there’s wide agreement that effective benchmarking tools are needed and will help drive quantum technology advances and eventual deployment. But getting them right is complicated.
- At this stage of the industry’s development, virtually all existing quantum computers are developmental themselves and changing fast.
- There’s also a proliferation of qubit types upon which the systems are based that can lead to vastly different architectures; QCs based superconducting qubits, (e.g. IBM, Google), trapped ions (e.g. Quantinuum and IonQ), neutral atoms (e.g. QuEra and Atom Computing) and photonics (e.g. PsiQuantum and Xanadu) can physically be very different.
Building apples-to-apples metrics is challenging, particularly at what’s generally called the system level. Currently, there are a variety of benchmarks around, some focused on system performances characteristics such as Quantum Volume (QV), first developed by IBM. Others like Volumetric Benchmarking (VB), introduced by the Quantum Performance Laboratory (QPL) at Sandia National Laboratories, are intended to address some of QV limits. Others, such as Algorithmic Qubits (AQ), have been coined by individual companies, in this case IonQ, and emphasize applications but aren’t widely adopted by others.
Benchmarking based on application performance – rather than say system metrics such as gate fidelity or circuit layer operations per second (CLOPS) – solves this problem. Broadly, it abstracts away underlying system and architecture details and focuses on how fast and accurately a particular quantum computer runs an application/algorithm. Rival vendors and potential users should be able to make useful comparisons among different quantum computers.
QED-C, of course, is a natural place for benchmarking development, and its focus has long been on application-oriented benchmarking.
Here’s the abstract from the paper (with slight formatting change), which that does a nice job summarizing QED-C’s strategy and what’s new:
“The QED-C suite of Application-Oriented Benchmarks provides the ability to gauge performance characteristics of quantum computers as applied to real-world applications. Its benchmark programs sweep over a range of problem sizes and inputs, capturing key performance metrics related to the quality of results, total time of execution, and quantum gate resources consumed. In this manuscript, we investigate challenges in broadening the relevance of this benchmarking methodology to applications of greater complexity.
- “First, we introduce a method for improving landscape coverage by varying algorithm parameters systematically, exemplifying this functionality in a new scalable HHL linear equation solver benchmark.
- “Second, we add a VQE implementation of a Hydrogen Lattice simulation to the QED-C suite, and introduce a methodology for analyzing the result quality and run-time cost trade-off. We observe a decrease in accuracy with increased number of qubits, but only a mild increase in the execution time.
- “Third, unique characteristics of a supervised machine-learning classification application are explored as a benchmark to gauge the extensibility of the framework to new classes of application. Applying this to a binary classification problem revealed the increase in training time required for larger anzatz circuits, and the significant classical overhead.
- “Fourth, we add methods to include optimization and error mitigation in the benchmarking workflow which allows us to: identify a favorable trade off between approximate gate synthesis and gate noise; observe the benefits of measurement error mitigation and a form of deterministic error mitigation algorithm; and to contrast the improvement with the resulting time overhead.
“Looking ahead, we discuss how the benchmark framework can be instrumental in facilitating the exploration of algorithmic options and their impact on performance.”
It is noteworthy that the latest work relies almost entirely on so-called “noisy simulators” rather than running the benchmarks on actual quantum hardware. Noisy simulators are classical systems that emulate existing quantum computing technology and strive to capture the noise (error-producing characteristics) of existing systems to produce more realistic results.
One quantum researcher lauded the work, thought the suite of applications was solid, but was disappointed more work wasn’t performed on quantum hardware – “I have only looked at the manuscript briefly, but noted that many of the results are using numerical simulators. I was surprised by this given the number of hardware companies collaborating – shouldn’t results on hardware from those companies be key. This raised a question for me about what can be deduced about performance from benchmarking a simulated model that has not been validated.”
Two results figures shown below.
Presumably that will be forthcoming. Quantum computing benchmarking, like the machines themselves, is still at an early stage, and Merzbacher emphasized improvements and additions were coming to the QED-C benchmarking effort.
Early in the paper, the QED-C researchers emphasize the challenges.
“The choices made in converting an algorithm to a scalable and verifiable benchmark will have consequences on how the benchmark performs on quantum hardware. Many of these choices impact the number of two-qubit gates, which is the dominant source of error in most current generation systems, and therefore strongly correlates with the performance. Evidence of this can be seen in the QED-C suite where decisions made to simplify verification allow for effective compilation that substantially reduces the number of two-qubit gates as discussed below,” they write in the introduction.
“Additionally, the choice to have some circuits ideally generate computational basis states means results are greatly improved with plurality voting error mitigation techniques, which are known to scale poorly. Both of these options are unique to the benchmark version of the application and are not typical for an actual use case. It is important to have benchmarks that both represent application use cases and run with comparable resource requirements.”
Throughout this manuscript, the figure of merit used to represent the quality of result for individual circuits is the “Normalized Hellinger Fidelity”, a modification of the standard “Hellinger Fidelity” that scales the metric to account for the uniform distribution produced by a completely noisy device.
The paper concludes with: “Cloud-accessible quantum computers are attracting a wide audience of potential users, but the challenges in understanding their capabilities create a significant barrier to the adoption of quantum computing. The QED-C Application-Oriented Benchmark suite makes it easy for new users to assess a machine’s ability to implement applications, and its volumetric visualization of the results is designed to be simple and intuitive. In this manuscript, we described several enhancements made to this benchmark suite. This is an ongoing effort, established under the direction of the QED-C (Quantum Economic Development Consortium), with work organized and managed by QED-C members within the broad community of quantum computing system providers and quantum software developers.
“In enhancing the suite and developing new benchmarks, we have expanded the framework to offer greater control over the properties and configuration of the applications used as benchmarks. It makes it possible, not only to increase coverage of the execution landscape but to explore various algorithmic variations. One reason to do this is to determine whether there are certain applications or variants of them that perform better on a specific class of hardware. We anticipate that our work will facilitate the adoption of quantum computing, and encourage economic development within the industry.”
The paper is best read directly and the code used is “readily available at https://github.com/SRI-International/QC-App-Oriented-Benchmarks. Detailed instructions are provided in the repository.”
A link to QED-C paper can be found here.
For the Chart of Qubit Modalities from Computing Community Consortium Report, click here.
Acknowledgment Section of Paper
“The Quantum Economic Development Consortium (QED- C), a group of commercial organizations, government institutions, and academia formed a Technical Advisory Committee (TAC) to study the landscape of standards development in quantum technologies and to identify ways to encourage economic development through standards. In this context, the Standards TAC undertook to create the suite of Application-Oriented Per- formance Benchmarks for Quantum Computing as an open source project, with contributions from many members of the QED-C involved in Quantum Computing. We thank the many members of the QED-C for their valuable input in reviewing and enhancing this work.
“We acknowledge the use of IBM Quantum services for this work. The views expressed are those of the authors and do not reflect the official policy or position of IBM or the IBM Quantum team. IBM Quantum. https://quantum- computing.ibm.com/, 2023. We acknowledge Quantinuum for contributing the results from their commercial H1-1 hard- ware. We thank Q-CTRL for supplying the environment and performing the execution of several of the QED-C benchmarks.”