How will programming future systems differ from current practice? This is an ever-present question in computing. Yet it has, perhaps, never been more pressing given the rise of heterogeneous architectures and diverse hardware, the steady incorporation of AI technology, and the proliferation of new programming languages and models.
At SC21, a distinguished panel tackled this broad question. Higher levels of abstraction, a clearer focus on data movement – not compute functions – and the rise of domain-specific languages as important tools were among the dominant points of discussion, which touched on topics as diverse programming Cerebras’s wafer-scale chip to FPGAs.
Moderated by Hal Finkel (DOE), the panelists included Kathy Yelick (UC Berkeley), Saman Amarasinghe (MIT), Torsten Hoefler (ETH Zürich), Maya Gokhale (LLBNL) and Justin Gottschlich (Intel). Capturing the full discussion is too daunting, but each panelist made an opening statement that captures (at least directionally) much of their thinking. Presented here are brief portions (lightly edited) of panelists’ opening remarks.
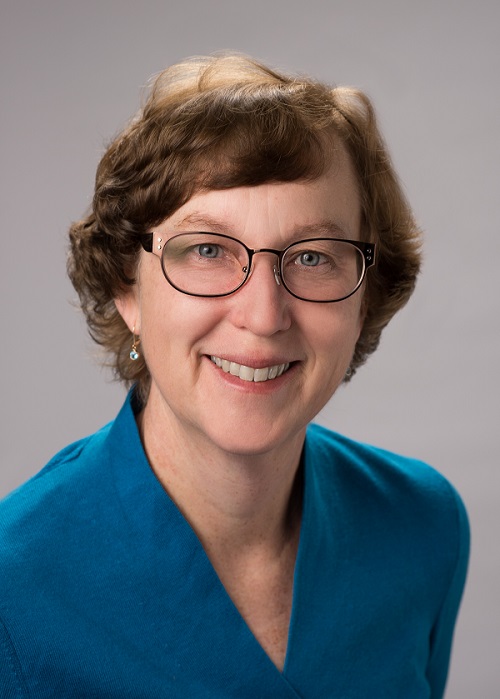
Yelick, who just assumed her new role as vice chancellor of research at LBNL, kicked off the panel saying, “[In] scientific computing, in general, I think we should think about how people are programming at much higher level of abstraction than we’re used to. I think if you look at machine learning, and the packages that people have built for machine learning, they’ve really shown that you can, with a lot of work in terms of how you implement some of those underlying algorithms, get very good performance out of those.
“That opens up HPC-type of access to a much broader community of people if they can program at the level of something like TensorFlow. And I’d like people to also think a little bit about systems like Julia and Jupyter notebooks as really the interface to the computers, rather than thinking about programming and languages based on things like C/C++ or Fortran. So really, I’m going to be advocating for a much higher level of abstraction, which is not to say that some of us won’t still be programming at a much lower level.”
Next up was Amarasinghe, who leads the compiler research group in MIT’s Computer Science & Artificial Intelligence Laboratory (CSAIL). A leader in the field of high-performance domain-specific languages, Amarasinghe’s group developed the Halide, TACO, Simit, and many other domain-specific languages and compilers,
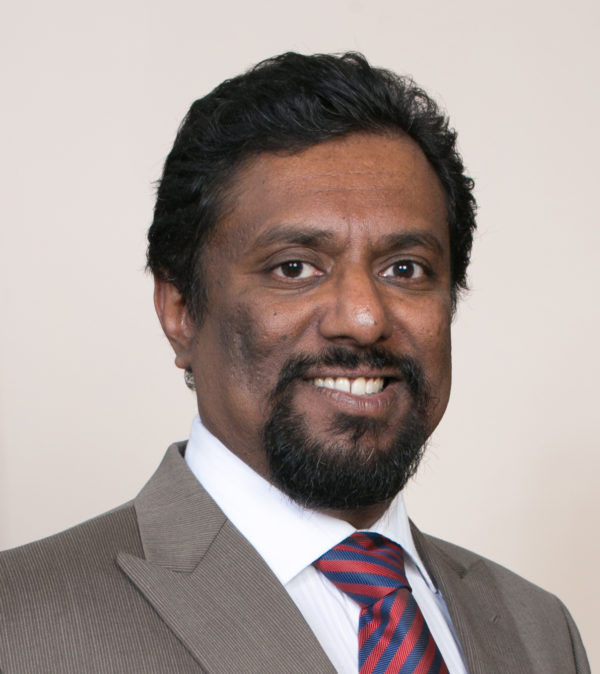
“If you think about domain-specific languages, [it’s] not too much of a stretch – even if you say you are a C programmer, or Fortran programmer or Python programmer – to say nobody writes loops and arrays and low level things in these languages. We all use libraries. All the systems are based on libraries and that means you’re already programming in higher level abstraction with one caveat. These libraries don’t have understanding of how the entire thing is connected together. So, when you call a library function, it’s a standalone thing; it will do what’s asked and return,” he said.
“What a domain-specific language or domain-specific compiler does is, it can figure out the control flow between these library calls, understand how these things get stitched together and use that to begin to optimize performance. This is especially important now and for the future, because memory systems and data movement are becoming a really important issue,” said Amarasinghe.
Perhaps the most forceful champion for focusing on data movement in future programming development was Hoefler, who directs the Scalable Parallel Computing Laboratory (SPCL) at ETH Zurich. He argued counting FLOPS, as is done in ranking the Top500, misses the point in modern computing.
Commenting on the use of new large models such as GPT-3, he said, “Many companies are spending 10s of millions of dollars to train these models, and these are real HPC problems. They are the largest models people have trained [and] very much [what] we care about. We actually analyzed the workload a little bit more in detail. We found that the 99.8 percent of the floating-point operations in this workload is actually comprised of Tensor contractions [and] Tensor contractions are all expressed as matrix multiplication.
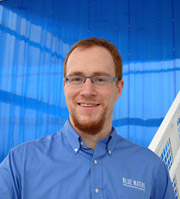
“So, this is wonderful, isn’t it? 99.8 percent of this workload is matrix multiplication. But if you actually look at the remaining 0.2 percent of operations in this workload, [it] turns out those are taking about 40 percent of the runtime. [That’s] because these Tensor contractions have been super highly-optimized over the years. The problem now [that] dominates everything else is data movement. We did some optimizations that I don’t want to go into detail about that show that you can actually speed this up quite significantly, and you can save millions of dollars by just looking at data movement,” said Hoefler.
Gottschlich, who is a principal AI scientist at Intel Labs and the director and founder of the machine programming research group at Intel, noted how Intel’s perspective on programing models has changed.
“When I joined back in 2010, Intel was very much a monolithic computing company, it was just a CPU. As I suspect everyone in the audience knows, we now consider ourselves to be very heterogeneous,” he said. “One of the core challenges we see today is not so much in the compute, but in the data movement. So, I just wanted to quickly acknowledge that I think the data movement, and figuring out how to deal with that, especially as we grow into deeper stochastic systems that tend to be improving their accuracy, as you have more IID data (independent and identically distributed data), that it becomes even more important that we figure out how to handle that that data movement problem.”
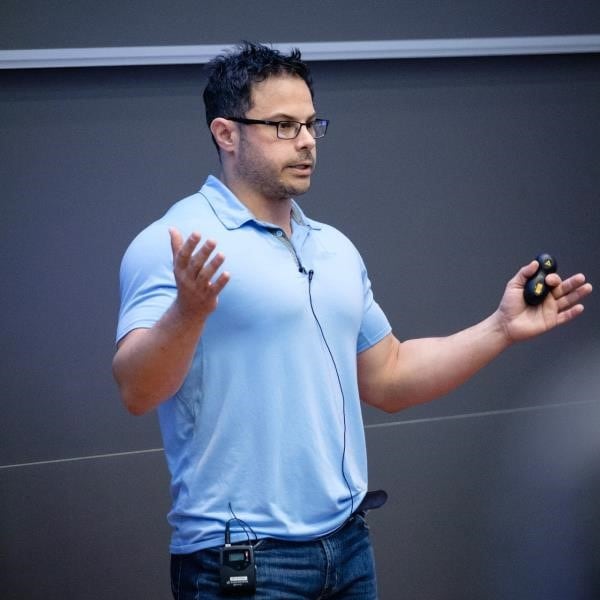
“Back in 2018, we published this paper, actually jointly with Saman (Amarasinghe) and some others, on the three pillars of machine programming. Machine programming is principally this idea that we are going to try to automate the development of software, and a byproduct of that is the automation of development of hardware given that much of hardware is developed through software. The three pillars are intention, invention and adaptation. Intention is principally concerned with trying to identify novel ways or improve the existing ways for programmers to specify their ideas to the machine. So, going back to, I think, both Kathy and Saman’s comments about higher order abstractions, and DSLs. In fact, I fully agree with this. I think that as we move forward, I suspect that to get outstanding performance, we really need to have this separation of intention from invention and adaptation. Once the intention is understood by the machine, then we can start to invent the algorithms and data structures that are necessary to fulfill that intention.”
Last to deliver intro remarks was Gokhale, distinguished member of technical staff at LLNL and an expert in reconfigurable computing and data intensive architectures.
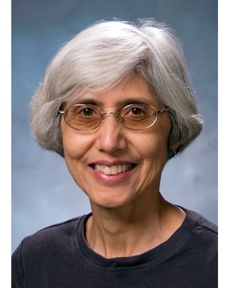
“I feel as if we’re in a fix right now with a fusion of programming models and it’s because of scaling laws, which we all know very well, between the feature size and the power. What we’ve done is build specialized widgets, that do a smaller thing, but do it very well rather than a general-purpose thing. That is a cause of a lot of problems. [It’s] one factor that is leading us to a lot of new ideas in programming models, this idea of specialization and putting heterogeneous pieces together,” said Gokhale.
“To me, the future is system-on-chip (OSC) like environments. So, heterogeneous compute models, data and or control-driven, tightly or loosely-coupled. [For example,] if you’ve worked for Apple or worked on cell phones, that SOC environment. I have a background in reconfigurable computing with FPGAs that is the combination of SOC-like environment and higher level programming. It’s a difficult environment to work in, but I see that’s where we’re going. On the other side, I see workflows for programming, [with] model interfacing and mapping. [Often] you think of your favorite DSL; it’s just so elegant and so mathematical. But it has to talk to other pieces of things and how do you make it do that? How do you interoperate? [L]arge HPC workflows have embodied some of those ideas of being able to interface with [DSLs],” she said.
A rich discussion followed the introductory comments and the SC21 video was still posted as of this writing and accessible by SC21 registrants.