Nvidia’s Hopper-generation H100 GPU is continuing its slow march toward “current-generation.” After Nvidia announced that the H100 was in “full production” last September, the chip made its formal debut in November with the Green500-topping Henri system. Now, at the first GTC of the year, Nvidia is touting the expanded reach of the H100 in the market. One of the flagship customers: Mitsui & Co., which is working with Nvidia on an H100-powered system called “Tokyo-1” that will be aimed at generative AI models for drug discovery.
Tokyo-1
Tokyo-1 will, initially, consist of 16 DGX H100 systems, each equipped with octuple H100 GPUs. By Nvidia’s AI flops math, this works out to around half an exaflop of AI power; as each H100 – there will be 128 to start – delivers 30 teraflops of peak FP64 power, it should work out to around 3.84 peak petaflops. The system will be operated by Xeureka (a subsidiary of Mitsui & Co. focused on AI drug discovery). Nvidia expects the system to become operational this year, and there are plans to expand the system with additional nodes.
More telling than the hardware is the purpose. Where previous years might have seen mentions of neural networks around similarly targeted systems, Nvidia’s announcement of Tokyo-1 touts it, instead, as the “first generative AI supercomputer for [the] pharmaceutical industry,” capitalizing on a zeitgeist dominated by ChatGPT and other generative AI tools.
The system will support AI-powered drug discovery for Japan’s large pharmaceutical industry (Nvidia describes it as the third largest in the world). By way of example, Nvidia mentioned generative AI models for novel molecular structures and large language models for analyzing protein and DNA data (powered by Nvidia’s BioNeMo drug discovery software). This isn’t all the supercomputer will be useful for, of course – Nvidia says it will be used for tasks like high-resolution molecular dynamics simulations and quantum chemistry, and the system will also be used to support medical device companies.
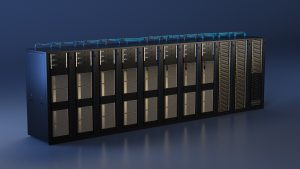
“Japanese pharma companies are experts in wet lab research, but they have not yet taken advantage of high performance computing and AI on a large scale,” said Yuhi Abe, general manager of the digital healthcare business department at Mitsui. “With Tokyo-1, we are creating an innovation hub that will enable the pharma industry to transform the landscape with state-of-the-art tools for AI-accelerated drug discovery.”
The project already has buy-in from several large Japanese pharma companies that plan to use Tokyo-1 for drug discovery projects. “Training AI models requires significant computational power, and we believe that the massive GPU resources of Tokyo-1 will solve this problem,” said Hiromu Egashira, director of the Drug Discovery DX Office in the Drug Discovery Technology Department at Ono Pharmaceutical. “We envision our use of the DGX supercomputer to be very broad, including high-quality simulations, image analysis, video analysis and language models.”
… and the rest!
The biggest availability benchmark for the H100 today is cloud availability: Amazon, Microsoft and Oracle (among other cloud providers) are debuting the H100 on their cloud platforms, with Nvidia touting AWS’ “UltraClusters” that can scale up to 20,000 H100 GPUs. Nvidia shared early access cloud customers, too: Stability.ai will use AWS-hosted H100s to accelerate their text-to-image generative AI, and Anlatan will use CoreWeave-hosted H100s to accelerate text-to-image generative AI and AI-assisted story writing for their NovelAI app.
Most notable among the early cloud customers: OpenAI, creator of ChatGPT. Nvidia touted OpenAI’s plans to use Azure-hosted H100s to power its ongoing generative AI work (the company currently uses A100s). “The computational power of the Nvidia H100 Tensor Core GPU will be vital for enabling our efforts to push the frontier of AI training and inference,” said Greg Brockman, president and cofounder of OpenAI. “Nvidia’s advancements unlock our research and alignment work on systems like GPT-4.”
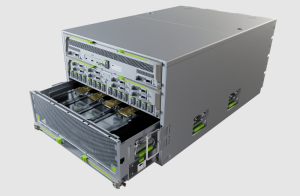
Outside of the cloud, Nvidia highlighted Meta’s adoption of the H100, which was telegraphed last October at the OCP Summit with the unveiling of Meta’s “Grand Teton” supercomputing platform design. Each 8U Grand Teton chassis holds up to eight H100s, with two of those chassis in an OCP rack. Meta told us that they have indeed deployed Grand Teton racks to form AI training clusters for “early adopters” and highlighted the ability of the design to scale out to thousands of interconnected GPUs – though it’s likely they haven’t yet realized those massive next-gen clusters, which last fall they targeted for a 2025 debut.
The DGX H100 is taking the “in full production” baton from its constituent GPU, with Nvidia CEO Jensen Huang announcing that the systems will be arriving soon. Before that broader availability, the first wave of DGX H100 deliveries will go to customers like Mitsui & Co., the KTH Royal Institute of Technology (for training large language models) and the Applied Physics University at Johns Hopkins University (also for training large language models).
Notably absent from today’s major announcements: updates on some of the major systems we’re expecting to use Hopper-generation hardware. This is pretty understandable vis-à-vis systems like Venado (Los Alamos National Laboratory) that will use the Grace Hopper Superchips, which weren’t discussed much during this GTC (there is, however, a GTC session scheduled for tomorrow on the infrastructure for Alps, which will leverage the Grace Hopper Superchips). That said, we were a bit more surprised to not hear updates on MareNostrum 5 – which will heavily feature Hopper GPUs – or, most surprisingly, Nvidia’s own Eos system, which was announced a year ago almost to the day. That system is set to include a total of 576 DGX H100 systems (4,608 H100 GPUs, for around 138 peak FP64 petaflops) and was initially targeted to debut “a few months” after that March 2022 announcement; since then, we haven’t received any updates.