The rise of AI – machine and deep learning – in life sciences has stirred the same excitement and same ‘fits-and-starts’ reality as elsewhere. Today, AI is mostly used in two areas: 1) embedded in life science instruments such as cryo-electron microscopes where it assists in feature recognition and lies largely hidden from users, and 2) in high profile projects such as NCI-DoE’s CANcer Distributed Learning Environment (CANDLE) which has access to supercomputing capacity and plenty of AI expertise. AI is, of course, being used against COVID19 at many large labs. There’s not much in between.
The truth is that AI remains in its infancy elsewhere in life sciences. The appetite for AI use is high but so are the early obstacles – not least the hype which has already ticked off clinicians, but also uneven data quality, non-optimal compute infrastructure, and limited AI expertise. On the plus side, practical pilot programs are now sprouting.
For this second part of the 2020 HPC in Life Sciences report HPCwire talked with Fernanda Foertter, BioTeam’s recent hire and now a senior scientific consultant specializing in AI. She joined BioTeam from Nvidia where she worked on AI in healthcare. Before that, Foertter was at Oak Ridge National Laboratory as a data scientist working on scalable algorithms for data analysis and deep learning. Her comments are blended with those from HPCwire’s earlier interview with Ari Berman, BioTeam CEO, Chris Dagdigian, co-founder, and Mike Steeves, senior scientific consultant.
Foertter sets the stage nicely:
TALE OF 2 DISCIPLINES – AI HUNGER IN RESEARCH NOT MATCHED IN THE CLINIC (YET)
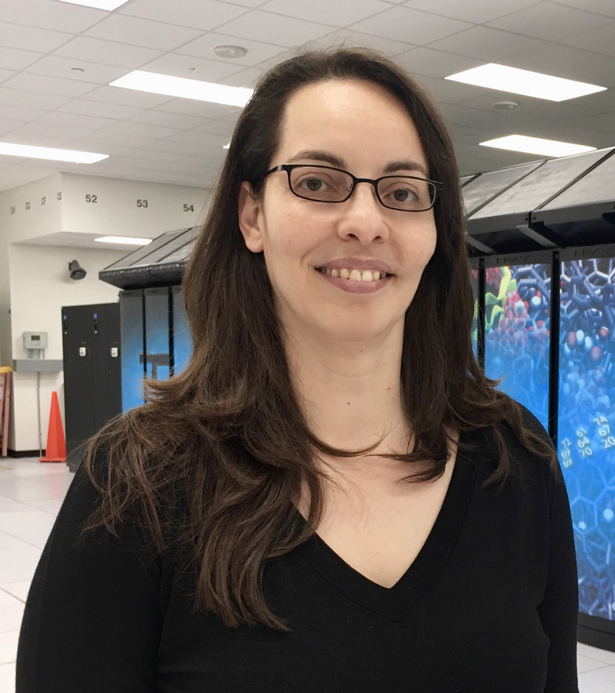
“Let’s talk a little bit on the historical side. If you look back at 2016, some of the life sciences related AI work that DOE was doing on supercomputers, we have the CANDLE project whose three pilots had the main basic AI applications in life sciences – so NLP (natural language processing) on SEER data; using AI for accelerating molecular dynamics; and drug discovery (drug screening). The first thing that really started to pick up was NLP. I know the NLP pilot is actually working really well today, [and] the molecular dynamics pilot is working really well too. The drug discovery didn’t have the right data to begin with and was delayed while in-vivo was being generated. “
“So those are the initial applications areas and as GPU memories grew larger, medical imaging started to mature within AI. These are the main areas in the life sciences today. where AI has been applied successfully – but still, all of these remain in the research realm. If you ask me, NLP is probably the easiest place that could go from research to production. Searching through text for context, for example giving people at least some idea that yes, something has been published before, or, we have something in this documentation here in our hospital, or some other patients’ file describes something similar, I think that these examples could actually work really well in the clinical setting.”
Also notable is that HPC, often with AI, has been used extensively in COVD-19 research (see HPCwire’s extensive coverage of HPC efforts fighting the pandemic). It seems reasonable to expect those efforts will not only bear fruit against COVID-19 but also generate new approaches for using HPC plus AI in life sciences research.
However, making AI work in the clinic has turned out to be challenging.
“When I went to Nvidia (Feb. 2018) the application that was most promising and fastest changing was on the medical imaging side. Even in the short, two years that I was there a lot changed. We went from talking about “can we discover pneumonia? can we discover tumors?” to talking about being able to grade tumors, which is much more refined. The one application I think everybody wishes would happen really, really quickly, but hasn’t really materialized is digital pathology,” said Foertter.
“The real-life pathology workflow is very difficult. Somebody, hopefully an experienced pathologist, has to go through a picture that has a very high pixel count, ie., very high resolution. They choose a few areas to investigate based on their experience because they cannot study the image in its entirety, and the pathologist will make a diagnosis. The miss rate is still somewhat absurdly high, especially for novice pathologists, anywhere between 30% to 40%. That means you can send somebody’s sample to pathology and depending on who sees it they’re going to miss that you have cancer or some other etiology. The reason [digital pathology] hasn’t really materialized is partly because the images are so large. To do any sort of convolution neural network on a really large image to train it would just break it. We were just doing the debugging of 3D convolutional neural networks for things like MRI reconstruction and digital pathology last year. It’s still, I think, not quite there. Just the memory size is really hard.”
Also problematic was the over-selling of AI’s capability to the clinician community replete with sometimes explicit claims clinicians would become obsolete.
“There has been a lot of animosity from physicians, justifiably so. The whole AI thing was kind of sold as if it could replace a lot of clinicians. And they [AI researchers] quickly corrected that, that kind of hubristic language,” said Foertter, who compares the situation to the initial reaction of airline pilots to fly-by-wire systems. “My brother flies a 737. He loves it because it’s not fly-by-wire. There’s still mechanical systems in there. That trust in fly-by-wire still hasn’t percolated through much of the pilot community. I feel the medical community will need to feel the trust and [be able] to investigate the data they don’t agree with or when they don’t understand the output. AI is still seen as a black box.”
The emerging picture is one of accelerating AI adoption in biomedical research but a slowness in the clinic that seems unlikely to change near-term. The latter seems driven by still nascent AI expertise, computational infrastructure shortcomings, and FDA requirements for underlying hardware and software used in the clinic. Foertter offers two examples:
- “One of the examples I like to use is Google’s project where they took fundus images – the back of the eye – and trained it to figure out certain things like cardiovascular risk and diabetes. They actually created an app out of the work and deployed it on their cloud. They collaborated with clinicians in Thailand who were enthusiastic, based on the initial results showing accuracy as high as 95% in diagnosis. But two years later Google realized that the 95% accuracy in development dropped substantially in the field, because number one, the quality of the images that it was trained on was much higher than Thailand had available coming out of their imaging equipment, and number two, the infrastructure wasn’t available for them to upload to the cloud when they had these [high quality image data] available.”
- The bulk of the [AI] talent and resources are located in Europe and US. These places have very stringent requirements, not in a negative sense but a safety and privacy sense, [for] hardware, software, and data. If you want to put a GPU, for example, in a medical instrument, which is an example that we had at Nvidia, by the time it goes to the FDA approval, the technology that was used to obtain regulatory approval was probably five years old? If you think about putting a 2015 GPU in the [approved] machine, that GPU number one is no longer in production, and number two the methodologies have changed.
Advances in AI technology and underlying methodologies are advancing too quickly for easy absorption into the clinic. Foertter and Berman both credit FDA efforts in trying to overcome some of these problems.
DOE’S TITAN SUPERCOMPUTER PRIMED THE AI PUMP
The reluctance to adopt AI in the clinic is completely reversed in biomedical research where there is something of a stampede building with enthusiasm for AI running rampant. Time will tell how the herd fares. BioTeam reports there’s a general lack of knowledge about basic AI-enabling technology and requirements along with uncertainty about picking projects.
Said Dagdigian, “Currently we are mostly enabling the ML/DL stuff from an IT infrastructure, you know, getting the systems up and running either on premise or in the cloud on helping them configure the storage, which needs to be higher performance. I will say that the people who can afford the DGXs seem to really love them, but other than that most of the people I’m working with are still on [CPU-centric systems]. They’re not picking the product as much they’re picking the toolkit or the environment. People are like, OK, we’re all in on TensorFlow and we’re going to start scaling up and training on that.”
Berman noted, “Some other things have started coming out like using AI frameworks to reduce the possibilities of drug targets [by assessing pocket binding characteristics matches] which is something that is being used massively at the moment in the coronavirus pandemic. That combined with electrodynamic simulations to verify that likelihood [of binding] is high and taking it into the lab to verify has the potential to reduce the time to drug development significantly, not to mention the cost.”
In terms of technology preferences, Berman said, “It really just depends on what the applications are. Most folks are just interested in having a small or large army of Nvidia Volta 100s in their arsenal and they can use several different generations of those just because that stuff gets a little expensive. Academics, who can rest on the non-production licenses, can use things like the Nvidia 1080 Ti or something like that. GPUs in general are still the preferred methodology for training, although there’s some recent innovations in the software stack.”
Foertter’s experience in high-end HPC, notably at ORNL, provides an interesting perspective on accelerator use (GPUs, specialty chips (e.g. Cerebras), FPGAs et al); this is especially so given Intel’s jump back into GPUs and AMD’s recent resurgence.
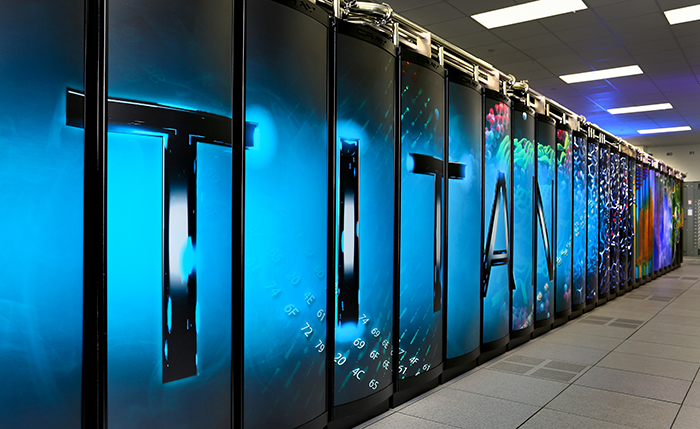
“Why is AI so popular now? Where did it begin? This resurgence began primarily because of HPC leveraging GPUs for scientific purposes. The same accelerated linear algebra libraries that are underneath the frameworks being used in AI were built because of Titan (DOE’s first heterogeneous GPU-centric supercomputer, ~2012). They were built for HPC and some bright folks realized they could leverage these for deep learning and all of a sudden AI explodes in 2014 – 2015. So, we’ve only been five years at building these math libraries and AI frameworks,” said Foertter.
“Now fast forward a year, around ~2016. Google says, well, we can probably build a special purpose TPU. They’re in the fourth generation of the TPU, and it still hasn’t quite taken off even within Google where there is still a dependence on GPUs. These specialty chips still require an underlying performant math library, and building these is not trivial. You can’t just kind of say, “Well, here’s a chip, it can do matrix multiply.” Okay, how do I integrate that into the framework? What libraries do I call?”
“I think there’s a good possibility that Intel, because Intel has had experience with building these HPC math libraries, can jump ahead even of AMD, even though AMD has also got the backing of DOE to create the same libraries for their GPU. Once those libraries exist for those newcomer GPUs, once AI frameworks have been built in a way that they can call any vendor’s version of BLAS for example, it’ll be fairly simple to run on emerging accelerators.
“I’m not sure that the special purpose stuff is really going to take off unless there’s somebody that really needs large models, very special models, you know, like the 3D convolutional nets; if a problem requires hardware that isn’t available from commodity options, it may be worth it for somebody to build it, sort of an FPGA style. But I don’t see those taking off and I don’t see a Google TPU taking off really, it’s too soon to tell,” said Foertter.
Not surprisingly the range of technology options available varies widely. DoE and NSF have big systems now and have been aggressively adding more (see NSF/PSC’s recent big bet on a Cerebras-based system). Big Pharma and big biotechs often have sophisticated systems, but mostly traditional CPU-centric architectures. Smaller biotechs and much of academia often have more limited computational resources. As a rule these days, a basic AI server has a couple of CPUs and 2-to-8 GPUs and virtually all major vendors have an AI line-up but even these are not cheap.
“The ability to put things on the cloud and burst to the cloud is a great place to try the new technologies and not have to worry about calling IT and racking it. I mean, I don’t know if you know about what it takes to rack up a DGX-2, but it takes a special team. It’s a big piece of equipment. You have to pay a team and they have to be certified to install that. It’s not something that you can kind of get off the shelf,” said Foertter. The cloud makes sense [when] there’s not a lot of IO, which is what usually costs a lot. If you’re in that scenario where there’s not a lot of IO, or you don’t have any other kind of legal constraints, where data can’t leave, or can’t stay, or HIPAA, the cloud is good place to get started.”
SHOULD AI’S HEAD BE IN THE CLOUDS?
The big cloud providers have all been adding accelerators of one or another ilk and adding (or developing) AI frameworks and integration tools. Berman notes Amazon’s SageMaker (ML development and deployment) is well integrated with other AWS services. Google, he said, also has powerful capabilities though they are somewhat more complicated to use.
The STRIDES Initiative from NIH, begun in July 2018 with Google and Amazon, is intended to help provide discounted access to cloud-services and various data repositories. Its benefits, as described by NIH include:
- Discounts on STRIDES¹ Initiative partner services – Favorable pricing on computing, storage, and related cloud services for NIH Institutes, Centers, and Offices (ICOs) and NIH-funded institutions.
- Professional services – Access to professional service consultations and technical support from the STRIDES Initiative partners.
- Training – Access to training for researchers, data owners, and others to help ensure optimal use of available tools and technologies.
- Potential collaborative engagements – Opportunities to explore methods and approaches that may advance NIH’s biomedical research objectives (with scope and milestones of engagements agreed upon separately).
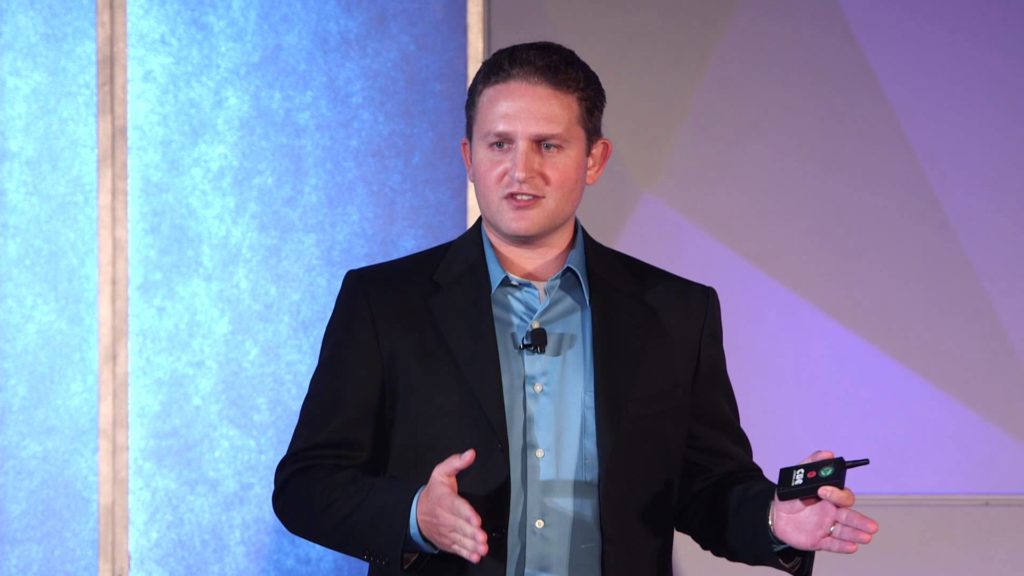
Said Berman, “STRIDES has generated broad interest for good reasons. There have been successful pilots with large datasets and large data platforms that NIH curates utilizing both GCP and AWS, and those have been really big wins. You know, STRIDES has been an integral part of the COVID-19 response that is going on right now and will continue to be. So I’d say as an initial release, especially for sophisticated groups, it’s been very positive. I think what’s coming down [in the future] is how to make it more accessible to people who don’t know how to operate clouds, and to implement platforms and things like that.”
Access to AI technology isn’t really the problem suggests BioTeam. It’s access to curated and integrated data that is a stumbling block. That and picking as starting (pilot) project. This is true even in big companies.
“Pharma companies generate tons of data. Some have been in the business for 40 years, like Johnson and Johnson or GSK. Along the way they’ve purchased 10, 15, 20 different Pharmas, and you’d be surprised at how many of those different acquired databases have never been integrated and the data are not harmonized. It’s amazing data, but it’s never been brought together. How do you use that? You need somebody that understands all these databases.”
Berman agreed. “As Fernanda laid out, data are so siloed. There are standards; there’s just 37 of them. Right? And they don’t talk to each other. There are entire consortia trying to figure this out. They’re trying to reduce the 37 to like eight, which still is too many. What I think is actually going to happen is that some of the existing standards are going to be come out stronger than others. Those standards are going to be used to create individual platforms that don’t talk to each other that are still siloed, so it has the effect of coalescing some of the data, but you still can’t mix the platforms. That’s what I think will happen. That also will enable a greater degree of AI and analytics to come in, but it’s still not going to realize the ability to query across all the data.”
Given the still fragmented and nascent world of AI, just getting started is currently the biggest challenge for many in commercial and academic life science research. Clinicians are a step or two behind them. While that sounds a bit formulaic and consultant-speak(ish), it also has the subdued ring of current reality. AI is still poorly defined. Machine learning and deep learning are concrete. But tools (hardware and s/w) for making practical use of ML and DL are emerging and now is likely the time to test the waters.
Said Foertter, “Realistically, they should be trying to do something. A lot of folks just don’t know where to start. They know that AI can bring them some value. They know they would like to explore to see if it could bring them value. But a lot of folks say they are just kind of waiting for somebody to sell them something. Here’s something you can put your data in and outcomes magic. That’s not what where are right now. That’s still probably another five years away to get to the point where people are going to be selling these sorts of ready-to-go, train-your-own solutions.”
BioTeam’s advice is to start small – a small pilot for which you have the data available and you have people that understand those data. Start with a small question, using a cloud resource or if you have adequate IT internal resources, and see where it goes.
Link to part one (HPC in Life Sciences 2020: the Rise of AMD, Taming Data Management’s Wild West), https://www.hpcwire.com/2020/05/20/hpc-in-life-sciences-2020-part-1-rise-of-amd-data-managements-wild-west-more/
Footnote 1 STRIDES is the NIH Science and Technology Research Infrastructure for Discovery, Experimentation, and Sustainability, https://datascience.nih.gov/strides