AI chip and systems startup Cerebras was one of many AI companies showcased at the AI Hardware Summit which concluded last week. Rather than dwell on its technology, which is widely known, Cerebras invited collaborators from Lawrence Livermore National Laboratory, Argonne National Laboratory and GlaxoSmithKline (GSK) to talk about their early work on Cerebras machines and future plans.
It was interesting for a change to hear of concrete activities involving one of the new class of emerging AI machines, and in Cerebras’s case, the activities were varied from nascent to quite far along.
First, some background. If you’re an AI watcher, no doubt Cerebras is a familiar name. Flush with cash and early customers, the Los Altos-based company has been an early success story. Its ‘chip’ – the wafer scale engine (WSE) – is enormous. It consumes 46,000 square millimeters of silicon and has 400,000 so-called Sparse Linear Algebra Compute (SLAC) cores with roughly 1.2 trillion transistors.
The cores are designed for sparse linear algebra primitives that underpin all neural network computation, says the company. The WSE also 18 gigabytes of on-chip memory, nine petabytes of memory bandwidth and 100 petabytes per sec of fabric bandwidth. Its big reveal took place at Hot Chips in 2019 (see HPCwire coverage).
Cerebras founder and CEO Andrew Feldman’s presentation included a slide showing the WSE next to Nvidia A100 GPU. “You (can) see our wafer scale engine. It’s the size of a dinner plate.” This is case where size clearly matters and the early users seem to agree. The WSE’s sheer size allows porting entire models, big models at that, onto the chip, which in combination with the design greatly reduces latency and speeds throughput.
Feldman was quick to emphasize Cerebras is a systems company – not a chip company – and as he put it, one wouldn’t build a Ferrari engine and put it in a Volkswagen. Cerebras’ first system is the CS-1, a 15 RU tall system (~26 inches) and you can get three in a standard rack, said Feldman (system specs).
The CS-1 system
The point of the panel was less a description of the Cerebras technology and more an effort to show the CS-1 being put to early, practical use. So: how’s it going?
LLNL Tackles Physics Simulation with CS-1
Let’s start with LLNL’s Livermore Computing Center (LCC). LCC already has substantial computing capabilities including its number two supercomputer, Lassen, which is an IBM-built system featuring Power9 CPUs, Nvidia V100s and InfiniBand fabric. It’s a 23-petaflops system with 44 racks that was #10 on the Top500 when first stood up and was #14 on the most recent Top500.
“So why am I here talking about Cerebras,” said Bronis de Supinski, CTO for LCC. “We have this vision for performing cognitive simulation using what we call a heterogeneous system architecture. Most supercomputers have the same node over and over. That is what the Lassen’s base architecture was. By adding the CS-1, we now have a volume that is specifically tuned and intended for running machine learning models.”
“We have integrated the CS-1 connecting it directly to Lassen on the InfiniBand fabric. I’d like to note our integration team has told me that siting was one of the smoothest sitings that they’ve been involved with, particularly for the first time a company has cited something on our machinery floor,” said de Supinski.
The goal is to build machine learning into physics simulations. “There’s a variety of places we can do this, from coordinating input to individual simulations, to the many time steps, or even very tightly integrated into the simulation and computing values that are used within every time step or every few time steps of the simulation. That’s what we’re looking to do,” he said.
Fast communication between the systems is critical. “Can we have the interaction between these different volumes of the system occur quickly enough? As we’ve already heard there’s quite a bit of bandwidth in and out of this system (CS-1). We’ve begun looking at this, and we’re actually building into a simulation called CRETIN[i] the ability to do some of the physics in every time step. What we’ve found is even though we need to ship a fairly large number of values into the CS-1 in order to bring back a fair number of values, that the inference happens quickly enough with the CS-1 that we can actually make this work,” said de Supinski.
“We’re also looking at additional things like being able to update models on the fly as we generate more data from other parts of the simulation, and also potentially running the detailed physics simulation to be able to have a more accurate model. Again, the capability the CS-1 provides us with being able to run these large-scale models is such that we believe that this will work,” he said.
GSK – It’s All About the Data Flood
By now, it’s a mantra that much of modern drug discovery is about sieving the data flood provided by modern experimental instruments. “To give you an illustration of the [problem], in the first quarter of this year, we generated more data than GSK had generated [during its] this entire 300-year history before. We need these AI methods to pull this data together to process it,” said Kim Branson, SVP, global head of AI and ML. “What’s happening in drug discovery is that AI methods can be used everywhere.”
Like most of biopharma, GSK has long relied heavily upon modern IT but only recently took a deep plunge into AI.
“Our AI team was established in 2019, and is currently distributed between San Francisco and London, our two main hubs, and Boston, Heidelberg, Philadelphia, and Tel Aviv. We [have] about 100 team members which means there’s a lot of people wanting a lot of CPU cycles. The unique nature of the problems we have, and the data sources and the size of the data sources led us to explore the world of custom deep learning silicon,” said Branson.
The rapid advance of functional genomics and the ability to sequence individual single cells, measuring the RNA and DNA content on those cells, is generating data at a massive scale. Moreover, the range of informatics being deployed – from varied statistical analysis to first-principle-like simulation – can all benefit from AI.
“We actually make predictions, we design experiments, we generate data, just purely to build a model and feed that back again. That’s our that’s a core tenet. We routinely feature [large and diverse] datasets such as medical images or pathology images,” said Branson noting Cerebras’s high data movement bandwidth was important.
Ease of use was also an issue. “When GSK was looking for novel computational platforms to really allow us to exploit the data we’re gathering, we did a survey of the custom computing landscape and Cerebras stood out as a company that actually had silicon you could use but more importantly also had a technological stack. That was also a key. They know how to take things in PyTorch and TensorFlow and actually deploy them,” Branson said.
ANL Gets Substantial Speedups with of CS-1
Argonne is one of the longest-term Cerebras collaborators. More generally, ANL is very active in AI research for the Department of Energy and has an active AI testbed on which ANL hopes to test as many different AI chips as it can. That said, ANL is actively using the CSI-1 on an array of ongoing projects, including recent COVID-19 research.
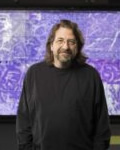
“We have over 100 AI projects at the lab,” said Rick Stevens, associate laboratory director for computing, environment and life sciences. “We’ve picked a couple of them to be our primary targets for working on the CS-1.” He briefly summarized four:
- LIGO. “This is a project that’s working on gravity wave detection [and] is building models that are suited for AI processing, the data coming off of things like LIGO to look for subtle signals of gravity waves. The project has been ongoing for about a year and is making good progress and achieving good speed ups on the CS-1,” said Stevens.
- Cancer Moonshot. “Probably our flagship project is cancer drug response prediction. This is a joint project between Argonne, the National Cancer Institute and collaborator institutions. We’ve been working on a series of models that run on the CS-1 predicting tumor response to drugs and these models are achieving speed ups of many hundreds of times on the CS-1 compared to our GPU baselines. We’re quite happy with these. These are models that are running on large scale machines across the different architectures,” Stevens said.
- COVID-19. “We’re also doing generative molecular design. This program is really aimed at generating new molecules that could be used as drugs. We’ve been applying this to our work in COVID-19. It’s also related to our cancer work in other areas where we’re trying to rapidly search through large spaces of drug molecules to find [candidate] molecules that meet certain criteria. Again, we’re getting great speedups there.”
- Dark Matter. “Finally, we have an active program in dark matter search. This is part of a large software activity, getting ready for the Large Synoptic Survey Telescope (LSST). With this application we’re trying to look for the signatures of halos around galaxies, the mass distributions that are indicative of dark matter halos. Gravitational lensing events are a classic example and we’re doing this both in simulated data and in observation,” he said.
One of the more interesting efforts by ANL is an early program to examine how easy it is (or isn’t) to use the CS-1.
“Most of our models are in TensorFlow, but some of them are coming in PyTorch. We feel pretty confident about the ease-of-use. This fall, [ANL director of data science and learning division] Ian Foster and I are opening up our CS-1 to about 35 graduate students who are coming in with a little bit of experience, but are going to be kind of fresh users. We’re going to use that [experience] to really kind of understand how easy it is for users to get on this machine and to build new models and to train.
“Now it’s still early in terms of the state of the software. There’s still layer types and features that we need and that we are collaborating on but the CS-1 is not that hard to use. If your teams are comfortable developing in TensorFlow or PyTorch, they’ll be right at home using this machine.”
ANL has more plans to aggressively use its CS-1. “Going forward, we have a large-scale program in AI for science at the lab and a rather novel strategy that we’re pursuing that will involve the CS-1 integrating advanced AI hardware with our laboratory robotic system [for] near-autonomous discovery in biology, chemistry, and materials science. So that’s what we’re looking forward to in the future. And we’re just extremely pleased with our collaboration,” said Stevens.
[i] CRETIN is a 1D, 2D, and 3D non-local thermodynamic equilibrium (NLTE) atomic kinetics/radiation transport code which follows the time evolution of atomic populations and photon distributions as radiation interacts with a plasma. It can provide detailed spectra for comparing with experimental diagnostics. https://wci.llnl.gov/simulation/computer-codes